5 Ways To P Value
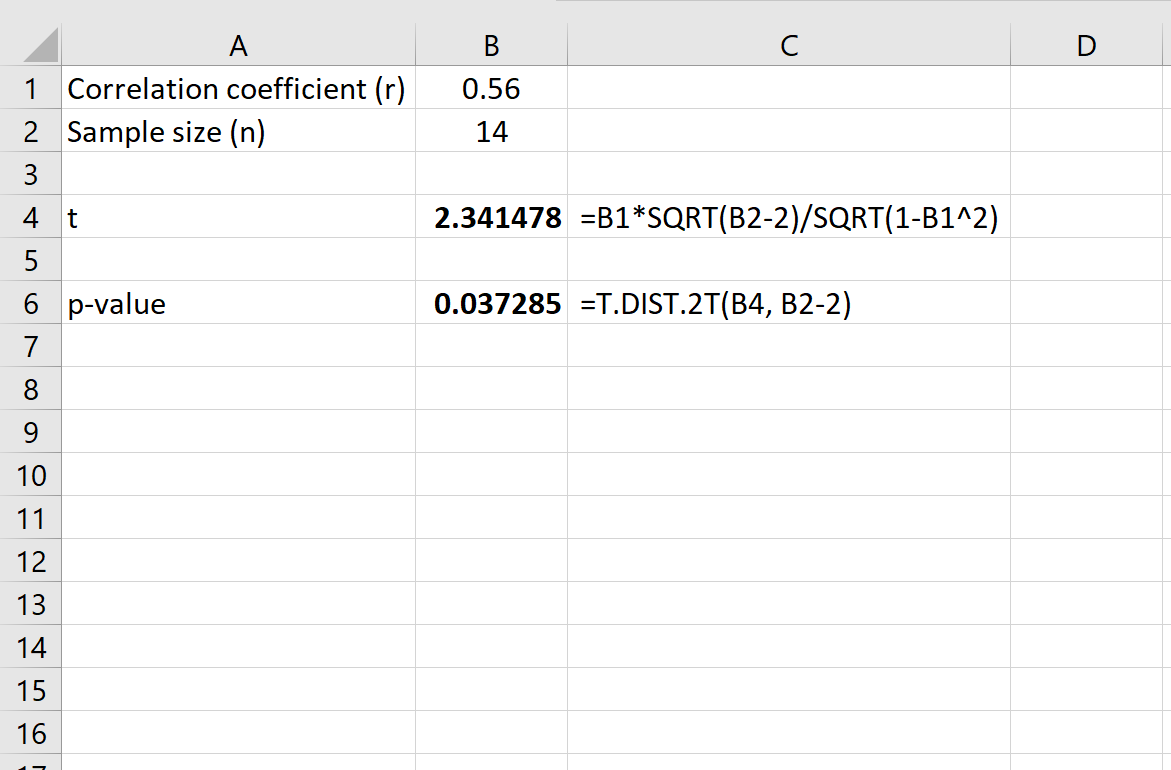
Introduction to P-Value
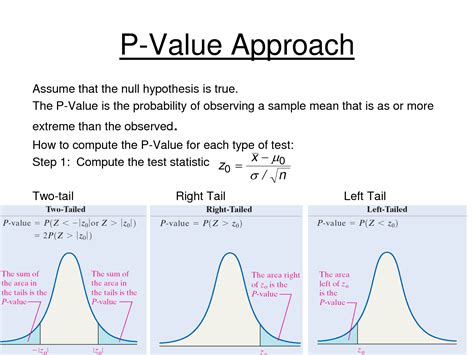
The p-value, or probability value, is a key concept in statistical hypothesis testing, representing the probability of observing results at least as extreme as those observed, assuming that the null hypothesis is true. The p-value is crucial in determining the significance of the results, helping researchers decide whether to reject the null hypothesis or not. In this article, we will explore five ways to understand and work with p-values, enhancing the interpretation and application of statistical analysis in research.
Understanding the Concept of P-Value
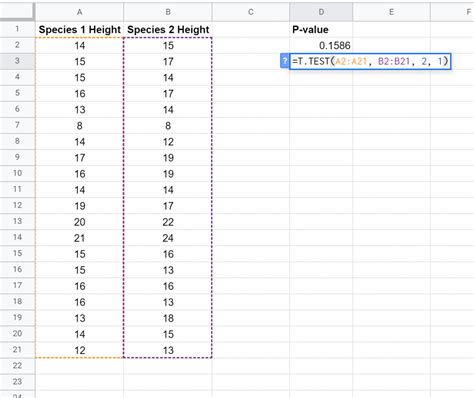
Before diving into the ways to work with p-values, itโs essential to understand what a p-value represents. The p-value is a number between 0 and 1 that indicates the strength of evidence against a null hypothesis. A small p-value (typically less than 0.05) suggests strong evidence against the null hypothesis, leading to its rejection, while a large p-value indicates weak evidence, suggesting the null hypothesis might be true.
1. Calculating P-Value

Calculating the p-value is the first step in hypothesis testing. This calculation depends on the type of test being conducted (e.g., t-test, ANOVA, regression analysis) and can be performed using statistical software or calculators. Understanding the formula and the assumptions behind each test is crucial for accurate calculation and interpretation of the p-value.
2. Interpreting P-Value Results
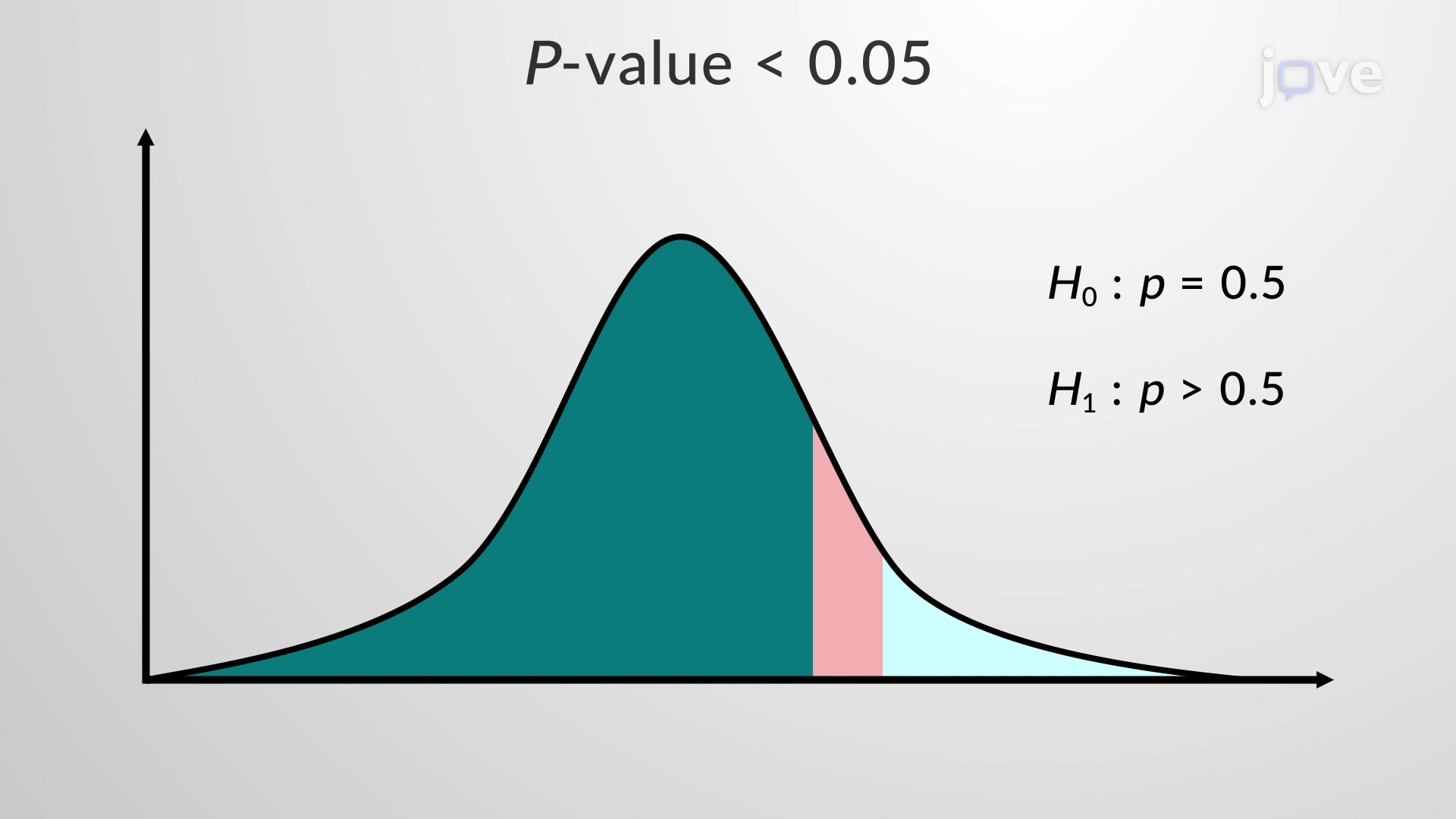
Interpreting p-value results involves comparing the calculated p-value to a predetermined significance level (usually 0.05). If the p-value is less than the significance level, the null hypothesis is rejected, suggesting that the observed effect is statistically significant. Correct interpretation of p-values is vital to avoid false positives or false negatives, which can lead to incorrect conclusions about the data.
3. Avoiding Common Misinterpretations

There are several common misinterpretations of p-values that researchers should be aware of: - Misunderstanding the p-value as the probability of the null hypothesis being true: The p-value does not indicate the probability that the null hypothesis is true; rather, it indicates the probability of observing the data (or more extreme data) assuming the null hypothesis is true. - Believing a non-significant result means there is no effect: A non-significant p-value does not necessarily mean there is no effect; it may indicate that the study lacked sufficient power to detect an effect. - Overlooking the importance of sample size and effect size: Small effects can be statistically significant with large sample sizes, and large effects may not be significant with small sample sizes.
4. Considering Alternative Statistical Measures
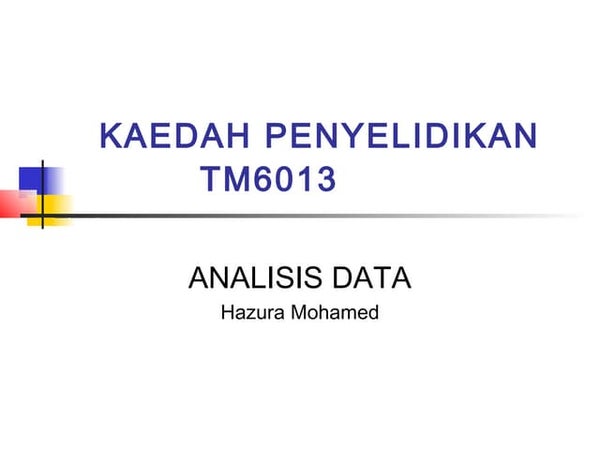
While p-values are widely used, they have limitations. Researchers should also consider alternative measures such as: - Confidence intervals: Provide a range of values within which the true population parameter is likely to lie. - Effect sizes: Quantify the magnitude of the observed effect, which can be more informative than p-values alone. - Bayesian methods: Offer an alternative approach to hypothesis testing that can provide more intuitive interpretations of evidence.
5. Integrating P-Values into Research Design
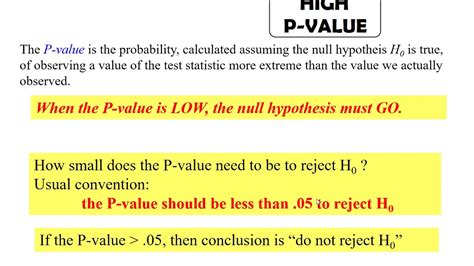
Finally, understanding how to integrate p-values into the research design is crucial. This includes planning for sufficient statistical power to detect expected effects, selecting appropriate statistical tests based on research questions and data characteristics, and interpreting results in the context of the research question. By doing so, researchers can ensure that their statistical analysis contributes meaningfully to the advancement of knowledge in their field.
๐ Note: Always consider the research context and the limitations of statistical methods when interpreting p-values.
In summary, working with p-values effectively involves understanding their calculation, interpretation, and limitations, as well as considering alternative statistical measures and integrating p-values thoughtfully into research design. By doing so, researchers can enhance the validity and reliability of their findings, contributing to a stronger evidence base in their field.
What does a p-value represent?
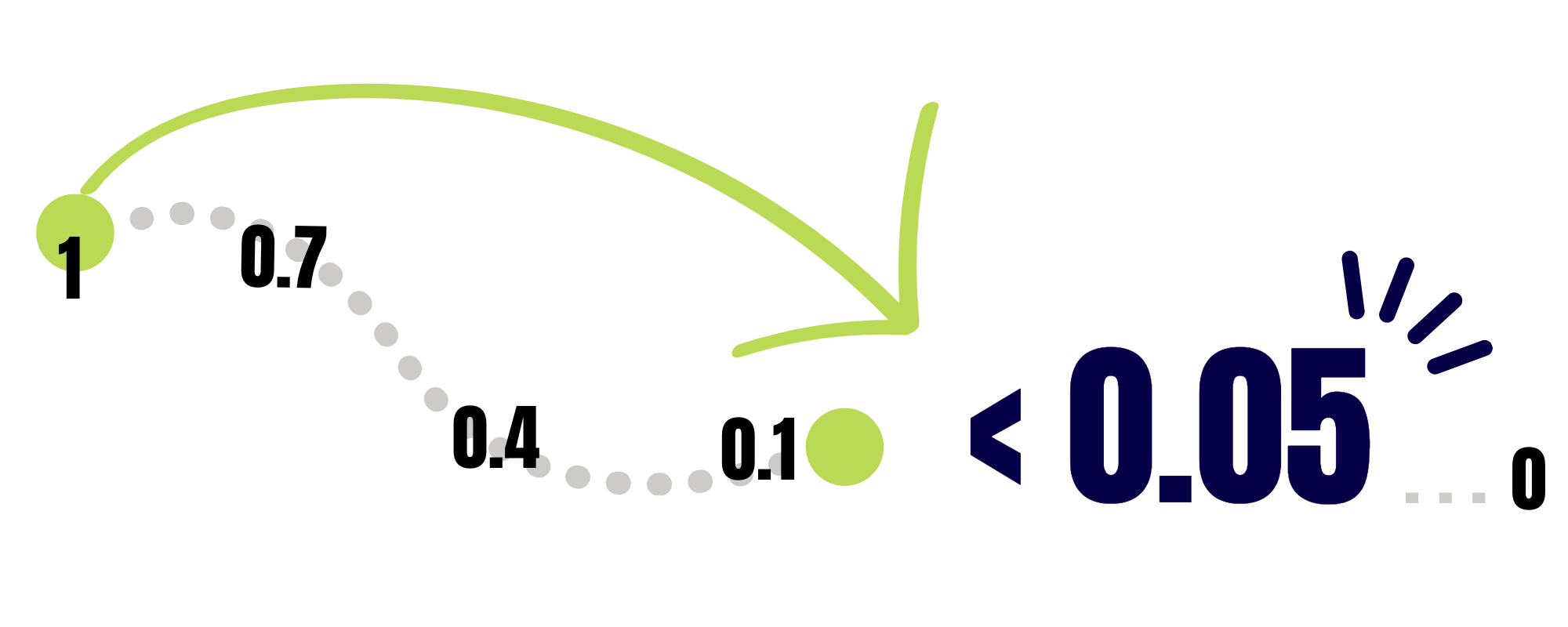
+
A p-value represents the probability of observing results at least as extreme as those observed, assuming that the null hypothesis is true.
How is the p-value calculated?
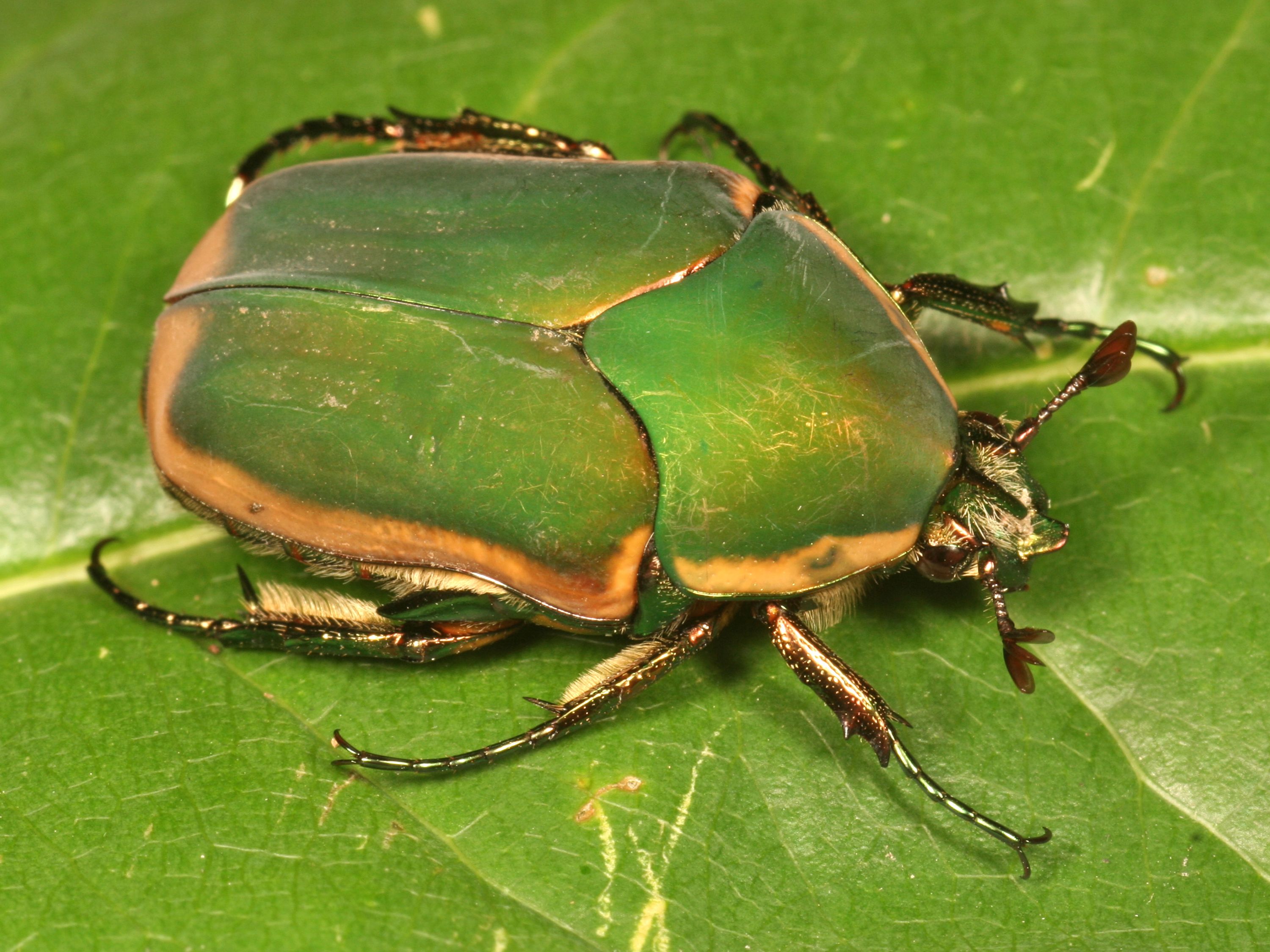
+
The calculation of the p-value depends on the type of statistical test being conducted and can be performed using statistical software or calculators.
What are common misinterpretations of p-values?
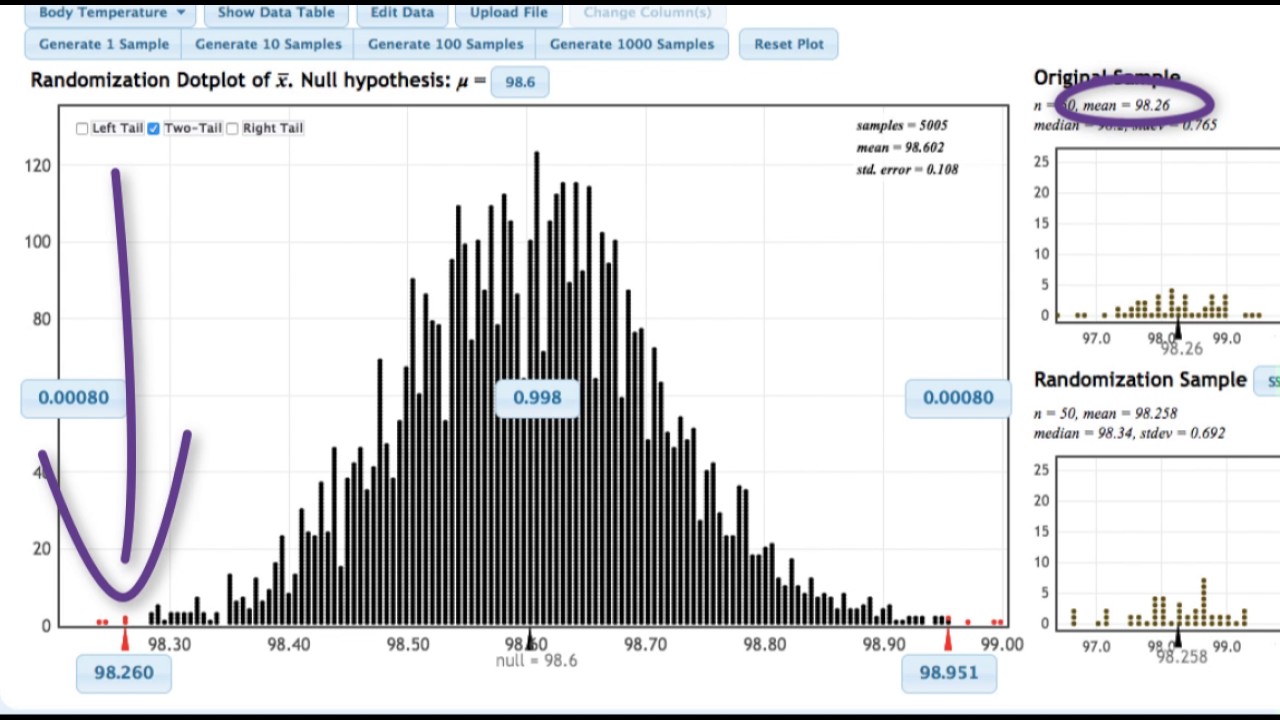
+
Common misinterpretations include misunderstanding the p-value as the probability of the null hypothesis being true, believing a non-significant result means there is no effect, and overlooking the importance of sample size and effect size.